Many wind energy companies are contending with unique challenges that were never a part of their emergency contingency plan. Employee safety is now synonymous with social distancing, supply chain disruptions are a matter of course, and the ability to transport technical experts for on-site repairs has been dramatically reduced.
However, today’s challenges have for the most part magnified existing maintenance challenges within wind farms, not introduced new ones. It was always a complex logistics operation to deliver equipment and people to the remote locations where wind farms are typically built. Maintenance crew safety is already at risk due to dangerous tasks that include electrical and lubrication issues or repairing turbines in high wind conditions.
For wind farms, this new reality is an opportunity to address maintenance challenges that may have been overlooked in the rush to meet demand and increase production.
Augmenting SCADA with AI-driven industrial analytics
SCADA and other types of condition monitoring form the basis of many predictive maintenance solutions. However, despite its ability to monitor large amounts of vibration, temperature, and velocity data, a SCADA approach is limited. Though SCADA monitoring does alert technicians that an asset is malfunctioning, this occurs only after manually set thresholds have been breached and the machine is already in distress. Similarly, SCADA is only capable of detecting an upcoming problem, and not pinpointing where the problem is or what might have caused this failure.
Wind farms are now looking to machine learning solutions to extend their analytics capabilities. Using the same sensors as SCADA, an AI-driven industrial analytics solution continuously captures big data from each asset. With automated machine learning, also known as AutoML, an AI algorithm assesses the data and chooses an optimal machine algorithm method for analysis, which in turn analyzes the data for abnormal machine behavior. Once a data aberration is detected, technicians receive an alert that the machine is developing a problem.
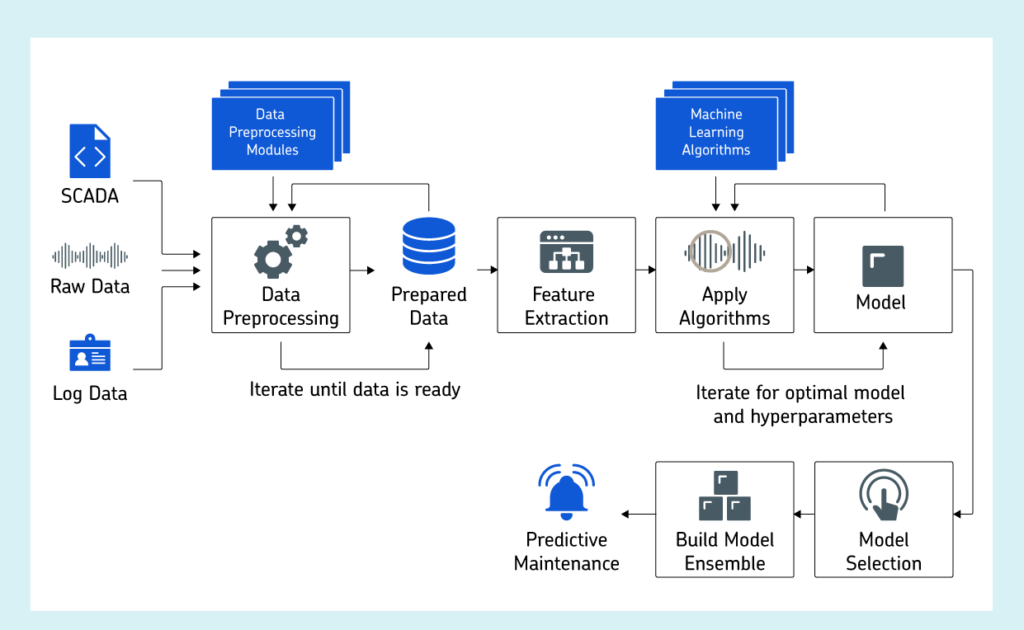
AutoML consequently augments condition-based monitoring in the following ways:
- Unlike SCADA, AutoML provides technicians with advance alerts about an emerging issue within the machine, long before manual thresholds are breached. With this timely warning technicians have the opportunity to schedule downtime, order parts, and fix issues before a shutdown occurs.
- AutoML not only collects big data but can attribute data to its respective sensors, giving technicians a more specific location of the unfolding machine issue.
- By comparing specific asset data with historical data culled from assets across the plant, AutoML can provide root-cause analysis and help technicians prevent the same malfunction occurring again.
At one wind farm that implemented AI, technicians were alerted that a turbine was experiencing pre-failure symptoms more than 50 hours before the shutdown was projected to occur. These type of advance warnings enable more effective O&M planning and scheduling and reduce the need for staff overtime and its associated costs.
Programming employee safety into wind farm maintenance
Wind farms do not regularly publicize maintenance team injury statistics. Certain risk factors are nevertheless associated with the remote location of most wind farms, including volatile weather conditions and increased distance from professional medical facilities. Other typical injuries relate to specialized maintenance tasks involving electricity or grease refilling which, to a less experienced technician, can be highly dangerous.
Compared to other energy industries, green sectors such as wind require a less hands-on monitoring approach to begin with. However, social distancing measures may still have an impact on wind O&M teams. While government-mandated guidelines are not the ideal catalyst for change, new requirements about how many people can be on-site at once and at what proximity can be the catalyst for hardwiring employee safety into your ways of working.
- Drones: Drones are increasingly taking on more routine maintenance tasks such as inspections and even basic repairs. In the UK, several robotic startups are developing drones specifically for wind farms that enable users to land drones on wind turbines.
- Remote monitoring: As the new normal sets in, remote monitoring has emerged as a compelling solution for protecting asset and human health. Connected technologies and remote diagnostic centers can provide near real-time insight into asset issues and reduce the number of maintenance personnel needed on the plant floor.
- Automated machine learning: Utilizing AI-driven industrial analytics within a remote monitoring solution is key to planning safer repairs, specifically enabling repairs teams to securely shut down and assets and loop in experts as needed.
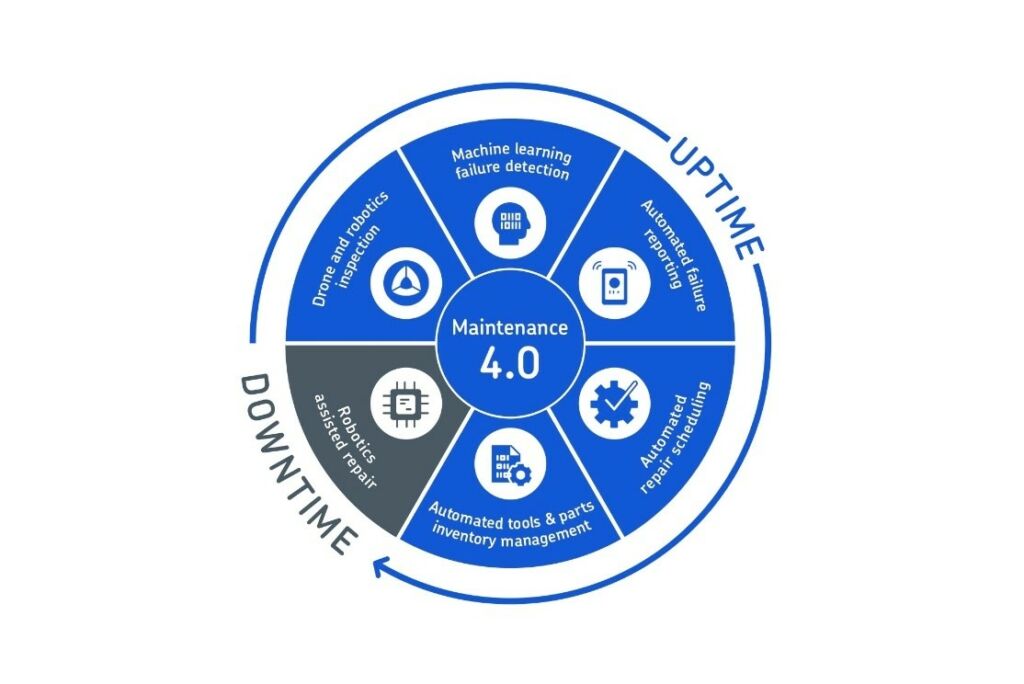
The shared safety goals of the above digitalization strategies are simple: to prevent injury and loss of life among wind maintenance repair crews.
Adapting to a changing supply chain
In April 2020, Stefan Gsänger, the secretary general of the World Wind Energy Association, urged governments to prioritize renewable energy sources: “Put investments in renewable energy, including wind power, at the center of your economic strategies. Not only will this help overcome the economic devastation caused by the corona crisis, it will also address the world’s second challenge, the climate crisis.”
Nevertheless, while wind energy is renewable and environmentally friendly, wind farm maintenance is often far from sustainable. A run-to-failure mindset means it is often simpler and cheaper for maintenance teams to purchase new equipment, rather than repair a severely damaged part.
As equipment suppliers, distributors, and customers adjust to the new normal, this will still be possible. In fact, it may become even easier. Deloitte argues that the pandemic will force customers to stop relying on siloed, linear supply chain methods and reduce risk by building a distributed, digital supply network.
At the time of writing, at least 35 percent of American manufacturers have reported experiencing some level of disruption in their supply chain. The continued existence of supply chains is critical for wind farms, just as it is for any industry. However, the present supply chain disruption invites wind farm executives to align plant operations with their product’s sustainability objective.
The average lifespan of a wind turbine is between 20 to 25 years, and it is inevitable that during that time there will be a need to order new parts. Nevertheless, wind farms can leverage predictive maintenance to extend asset lifespan and prevent or delay purchasing equipment in the first place.
The incremental savings opportunity
Green energy output and revenue are offset by maintenance expenses, which rise as assets age. Investing in an AI-driven industrial analytics solution can lead to long-term savings and revenue growth for a wind farm. Estimates suggest that 70 percent of wind turbine downtime is because of major repairs, and predictive maintenance’s ability to detect subtle machine behavior anomalies enables repair crews to find and fix problems long before they become major. Reducing wind turbine downtime will therefore not only increase wind energy output but minimize O&M costs as well.
A less obvious expense is inventory carrying costs. Once a plant has extra parts in its inventory, these parts must be reported and taxed as CAPEX. Though there is no specific data for inventory carrying costs in wind plants, as a rule these listless parts can cost plant owners between 15 and -25 percent of their worth in taxes per year. This unnecessary expense can similarly be mitigated with predictive maintenance. By giving technicians earlier warning about upcoming asset health failure, spare parts can be ordered on an as-needed basis and prevent the ordering of unneeded parts.
Though this latter cost-cutting benefit may be hampered by current supply chain complications, AI-driven industrial analytics’ reduction in unscheduled downtime offers significant financial benefits to wind farms.
What’s next for wind farms
The digitalization of maintenance is only one strategy for increasing sustainability, safety, and savings at wind farms. Other initiatives focus on automatic lubrication, which reduces the need for dangerous grease re-fillings and the less eco-friendly purchase of grease for wind turbine bearings. Some changes may also be made in terms of how maintenance crews work and changeover, regardless of the technologies they are utilizing.
However, within the recent economic and logistic uncertainty Maintenance 4.0 has shifted from a potential benefit to a concrete strategic tool. It is not about weathering the storm, or even about emerging stronger on the other side. Instead, it is about leveraging today’s unusual circumstances to catalyze maintenance changes within the plant for lasting asset health and output growth.